Staff Profile
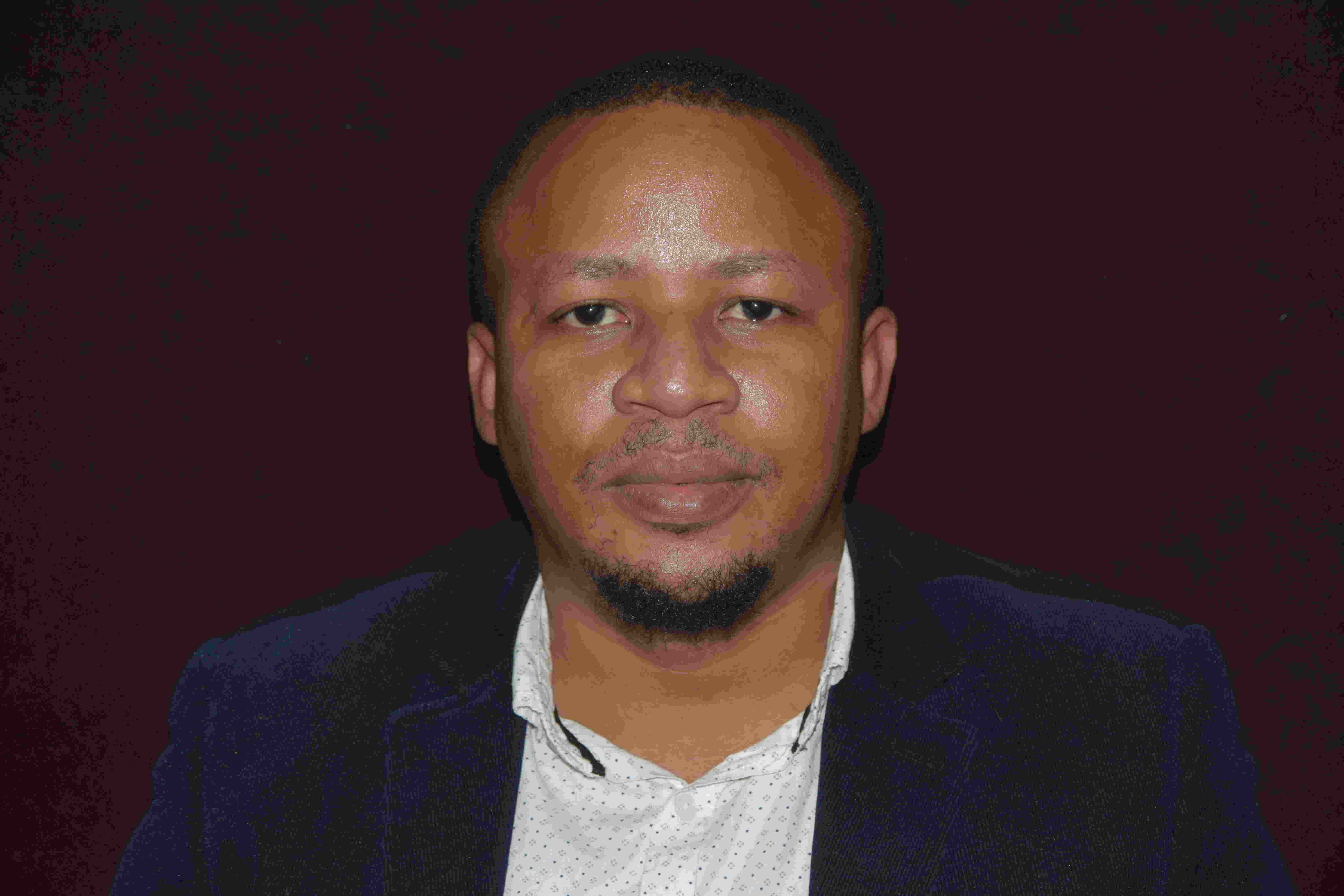
BIO
Isack E. Bulugu is a Tanzanian academic, researcher, and engineer whose career blends a strong foundation in science and technology with a deep commitment to education, innovation, and social impact. He currently serves as a Senior Lecturer in the Department of Electronics and Telecommunication Engineering at the University of Dar es Salaam, College of Information and Communication Technologies (CoICT), where he teaches, supervises postgraduate students, and leads research in emerging areas of computing and communication systems.
Dr. Bulugu’s fascination with electronics and computing began early in life, driving him to pursue higher education and advanced research in the fields of electronics and telecommunication Engineering. He holds a Ph.D. in Information and Communication Engineering from the University of Science and Technology of China, where he conducted pioneering work on feature extraction techniques for hand gesture recognition. He also earned an M.Eng. in Signal and Information Processing from Tianjin University of Technology and a BSc in Electronic Science and Communication from UDSM.
Dr. Bulugu has received numerous prestigious fellowships and awards throughout his academic journey. These include the CAS-TWAS President’s Scholarship, the Chinese Government Scholarship, the Talented Young Scientist Program (TYSP) Fellowship (2022–2023), and most recently, the University Administration Support Program (UASP) Fellowship in Research Management (2024–2025), USA. He is also a SPARX Program Fellow (2024–2026) under IEEE at Chalmers University of Technology, Sweden.
A prolific researcher, Dr. Bulugu has authored and co-authored over 15 peer-reviewed publications in international journals and conference proceedings. His research focuses on computer vision, machine learning, digital image processing, pattern recognition, and human-computer interaction, AI, Robotics. His work addresses both theoretical challenges and real-world applications, such as developing assistive technologies for students with disabilities and implementing smart systems in industrial settings.
In addition to his research, Dr. Bulugu is passionate about capacity building and mentorship. He has supervised numerous undergraduate and postgraduate theses. His commitment to community service is reflected in his involvement in STEM education outreach, both in Tanzania and through global academic exchange programs.
Dr. Bulugu is a Registered ICT Professional with the Information and Communication Technologies Commission (ICTC) and a Registered Electronics and Telecommunication Engineer with the Engineers Registration Board (ERB) of Tanzania. He is also an active member of several professional bodies, including the IEEE Robotics and Automation Society.
Beyond his professional accomplishments, Dr. Bulugu is known for his humility, discipline, and commitment to continuous learning. He enjoys engaging in intercultural dialogue, supporting community development projects, and exploring innovative teaching methods that bridge global knowledge with local relevance.
Through his scholarly contributions, leadership in education, and dedication to social impact, Dr. Bulugu stands out as a visionary scholar and an ambassador for academic excellence and collaboration in Africa and beyond.
RESEARCH
Real-time 3D Human Pose Estimation based on kinematic model
- 3D human pose estimation is used to predict the locations of body joints in 3D space. Besides the 3D pose, some methods also recover 3D human mesh from images or videos. This field has attracted much interest in recent years since it is used to provide extensive 3D structure information related to the human body. It can be applied to various applications, such as 3D animation industries, virtual or augmented reality, and 3D action prediction. 3D human pose analysis can be performed on monocular images or videos (normal camera feeds). Using multiple viewpoints or additional sensors (IMU or LiDAR), 3D pose estimation can be applied with information fusion techniques, which is a very challenging task. While 2D human datasets can be easily obtained, collecting accurate 3D pose image annotation is time-consuming, and manual labeling is not practical and expensive. Therefore, although 3D pose tracking has made significant advancements in recent years, especially due to the progress made in 2D human pose estimation, there are still several challenges to overcome: Model generalization, robustness to occlusion, and computation efficiency.
- 3D Human Body Modeling In human pose estimation, the location of human body parts is used to build a human body representation (such as a body skeleton pose) from visual input data. Therefore, human body modeling is an important aspect of human pose estimation. It is used to represent features and key points extracted from visual input data. Typically, a model-based approach is used to describe and infer human body poses and render 2D or 3D poses.
- Most methods use an N-joints rigid kinematic model where a human body is represented as an entity with joints and limbs, containing body kinematic structure and body shape information. Kinematic Model, also called the skeleton-based model, is used for 2D and 3D pose estimation. This flexible and intuitive human body model includes a set of joint positions and limb orientations to represent the human body structure. Therefore, skeleton pose estimation models are used to capture the relations between different body parts. However, kinematic models are limited in representing texture or shape information.
Real-time Hand Gesture Recognition/Sign Language Recognition based on Machine Learning models for Human Computer Interaction
Hand gesture recognition for human computer interaction, being a natural way of human computer interaction, is an area of active research in computer vision and machine learning. This is an area with many different possible applications, giving users a simpler and more natural way to communicate with robots/systems interfaces, without the need for extra devices. So, the primary goal of gesture recognition research is to create systems, which can identify specific human gestures and use them to convey information or for device control. For that, vision-based hand gesture interfaces require fast and extremely robust hand detection, and gesture recognition in real time.
Sign Language is a form of communication used primarily by people hard of hearing or deaf. This type of gesture-based language allows people to convey ideas and thoughts easily overcoming the barriers caused by difficulties from hearing issues.
A major issue with this convenient form of communication is the lack of knowledge of the language for the vast majority of the global population. Just as any other language, learning Sign Language takes much time and effort, discouraging to from being learned by the larger population.
However, an evident solution to this issue is present in the world of Machine Learning and Image Detection. Implementing predictive model technology to automatically classify Sign Language symbols can be used to create a form of real-time captioning for virtual conferences like Zoom meetings and other such things. This would greatly increase access of such services to those with hearing impairments as it would go hand-in-hand with voice-based captioning, creating a two-way communication system online for people with hearing issues.
Cloud Platform Construction for the Environmental Awareness of Internet of Things (IOT) system
Building upon existing industrial pollution treatment equipment, such as integrated sewage treatment systems, sensors, global positioning systems (GPS), and video surveillance technology can be integrated to establish a network for industrial pollution treatment. This network will allow for the real-time collection of environmental quality information, enabling the creation of a comprehensive, multi-level ecological monitoring network with full coverage.
To achieve this, sensor technology, wireless network technology, video recognition technology, and the Internet can be employed to develop a Cloud Platform for Environmental Awareness of the Internet of Things (IoT). This platform will enable the real-time dynamic perception of environmental quality.
Additionally, an environmental protection IoT data transmission system can be implemented by constructing both wired and wireless data paths to upload sensor and terminal monitoring data to the management platform. The overarching goal of this endeavor will be to achieve full intelligence in environmental protection businesses, including environmental quality monitoring, supervision, law enforcement against environmental pollution, and management decision-making. This will be made possible through the utilization of massive data resource service platforms.